Solving the Cash Flow Forecasting Problem
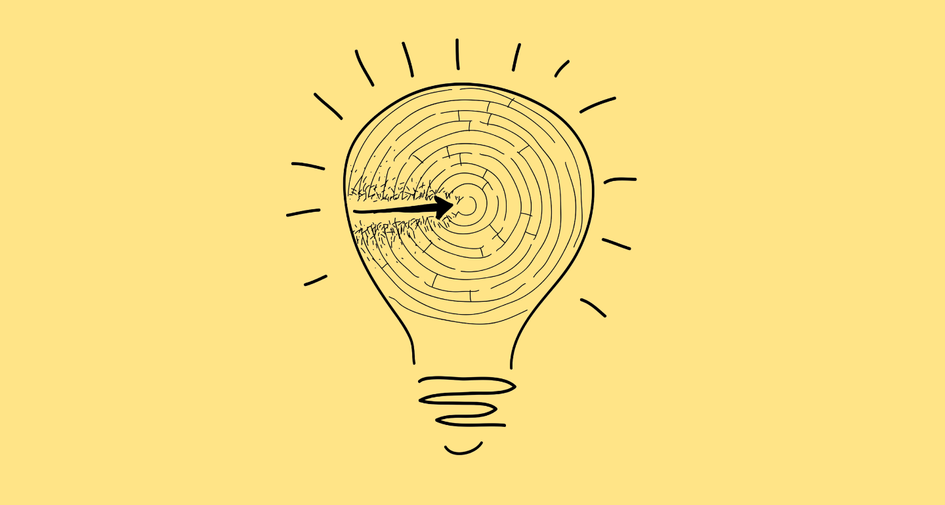
Many companies still have difficulty gaining deep and real-time visibility into the cash and working capital they need to survive and thrive. This can lead to sub-optimal decisions, sitting on too much idle cash, delayed reactions cash flow issues, and a range of other problems. According to Carlos Vega [Founder, Tesorio], “You need to understand cash flow to understand working capital, and since all businesses are becoming increasingly agile, cash must flow at the pace of business.” Vega argues, impacts on cash flow should inform every decision that is made from fine-tuning AR processes to timing payments, to making investments of all kinds. But that’s impossible without visibility into cash, a task that is made much harder due to the widespread over-reliance on static tools like spreadsheets and tedious and time-consuming processes to build cash forecasts.
Historically, it has been difficult to forecast cash. Many forecasts are primarily budgeting, using indirect forecasting techniques based on balance sheets and cash flow statements. Direct method forecasts, which are more relevant to cash flow and real-time business operations, are time-consuming to build and maintain, so are often out of date before they are even produced and can be acted upon.
“The Tesorio cash flow performance platform is a hybrid of the two techniques”, explains Vega. “By unwinding the general ledger in the right way, we can automate the actuals”, removing much of the pain associated with direct method cash flow forecasting. The platform then uses machine learning and AI to not only create real-time cash flow forecasts, but also visualize trends, run scenarios, and identify and address variances.
The way Tesorio uses the General Ledger data is central to its operation. In a traditional forecast, someone in finance has to identify which customers are outstanding. If the company has transacted with any of them in the past, the same individual has to then calculate their customers’ behavior patterns to assess how likely, and when, they are to pay into the future, given what’s outstanding. All this takes time.
“Tesorio automates this manual process using machine learning,” explains Vega. The ML works by analyzing all the metadata linked to each individual transaction within the forecast. This allows finance to right-click a particular transaction in the user interface and bring up all the relevant data (the customer, the invoice, the payment due date) behind the cash flow. With all the background information, finance can analyze how best to manage the impact of cash flow. “By connecting the accounting to other systems – sales, billing, and procurement – you can know what each cash flow is for, and you can make it actionable”.
Any changes to this data, such as an updated payment date provided by a customer, can be input to the system, automatically updating the forecast in real-time. The same platform is also used to streamline related tasks, so collections can, for example, create campaigns to send out emails to customers according to certain, predetermined conditions.
Critically, while Tesorio replaces the mundane processes, it does not, and cannot, replace finance’s knowledge of current and future events. “If there is a signal in the data, ML will highlight it for you. But there is no way a machine can know what you discussed with the board, or what you just discussed with your peers in sales and marketing about a new product”, explains Vega. This human knowledge can be easily incorporated into the forecast.
From the beginning, Tesorio’s use of ML means clients have an actionable forecast, but users can easily amend individual figures within the forecast to reflect new or additional information. They can override a figure in the ML forecast and enter the number they choose, perhaps to update a promise to pay date. They can adjust a figure, perhaps by increasing it by a certain percentage. Or they can calculate the figure using a different formula, effectively mirroring a spreadsheet calculation. Any changes to the figures automatically update the forecast in real-time, meaning finance is always making decisions on the latest cash flow information. The key to the effectiveness of the platform is the human plus machine inputs and interactions.
“For us, the understanding of cash flow is the backbone to which we could append all the other modules to automate daily tasks – understanding FX exposures, automating AR processes, AP activities, and much more,” says Vega.